Transforming Motor Maintenance with Predictive Vibration Analytics
1/16/20252 min read
In today’s world, where technology drives operational efficiency, motors play an unsung yet vital role in powering industries, from manufacturing to urban water supply systems. The challenges of ensuring their uninterrupted operation, however, have spurred a revolutionary solution: Predictive Maintenance through Vibration Analytics. 🚀
The Promise of Predictive Maintenance 🌟
Motors are the heartbeat ❤️ of many critical systems, and their failure can cause severe operational and financial setbacks. Traditional maintenance strategies, like reactive and preventive maintenance, are now being overshadowed by Condition-Based Maintenance (CBM). At its core, CBM leverages cutting-edge tools such as vibration analysis 📈 to continuously monitor motor health, enabling proactive measures before issues escalate.
The project undertaken at the Tamar Central Government Office demonstrates the potential of predictive analytics in managing motor health efficiently. Over a 10-month period, data collected from vibration sensors enabled the development of an advanced, AI-driven maintenance system. This system integrates data analysis, deep learning models, and a user-centric dashboard 🖥️ to empower decision-making.
The Methodology Behind the Innovation 🧠
Data-Driven Insights 🔍
The foundation of the project was a dataset spanning 10 months, capturing high-frequency vibration time series data from motor sensors. Through robust preprocessing techniques, inconsistencies like missing data were addressed, reducing errors and creating a clean slate for analysis.
Advanced Analytical Approaches 🚀
Exploratory Data Analysis (EDA): Initial data explorations uncovered trends and correlations. For example, axial acceleration showed an inverse relationship with speed, providing clues about motor performance.
Frequency Domain Analysis: Algorithms like Fast Fourier Transform (FFT) helped isolate critical vibration frequencies, identifying anomalies in motor vibrations.
Feature Engineering and Predictive Modeling: By extracting actionable features such as peak-to-peak values 📊 and using machine learning models, the project identified patterns that signaled potential faults.
Dynamic Deep Learning 🤖
Incorporating Recurrent Neural Networks (RNNs) allowed the system to process sequential data effectively. This architecture adapted dynamically to real-time data, enhancing the predictive accuracy of motor health forecasts. ⚡
Interactive Dashboards 📊
A user-friendly, web-based dashboard brought the data to life, presenting real-time health metrics, predictive alerts, and visualizations. Technicians could monitor trends, identify anomalies, and act promptly using this intuitive tool.
Challenges and Innovations ⚙️
The project wasn’t without hurdles:
Data Inconsistencies: Missing or invalid data points were frequent, necessitating sophisticated handling methods like forward filling and sequence reconstruction. 🛠️
Computational Demands: Techniques like Dynamic Time Warping (DTW) required significant resources. Leveraging optimized algorithms and GPU acceleration minimized delays. ⏱️
Deep Learning Overfitting: Advanced techniques like dropout layers and checkpointing addressed overfitting challenges in RNN models. 📉
Future Directions in Vibration Analytics 🌐
While the project has established a robust framework, future enhancements could include:
Machine Learning-Enhanced Peak-to-Peak Analysis: By integrating forecasting models, this could become a proactive fault detection tool. 🔮
Optimized Algorithms: Transitioning to GPU-accelerated methods like soft-DTW will reduce processing time and improve scalability. 💡
Cloud-Based Dashboards: Deploying dashboards on cloud servers ensures accessibility across devices, making data more actionable for technicians on the go. ☁️📱
A New Era for Smart Infrastructure Management 🌆
The Tamar project exemplifies the transformative potential of vibration analytics in predictive maintenance. Beyond ensuring uninterrupted service, it significantly reduces operational costs, extends motor lifespans, and enhances sustainability. 🌿
As industries embrace smart solutions, vibration analytics stands out as a game-changer for motor health management. With continuous advancements in AI and machine learning, the road ahead is clear: a future where predictive maintenance is not just a tool, but a standard. 🌟🛠️
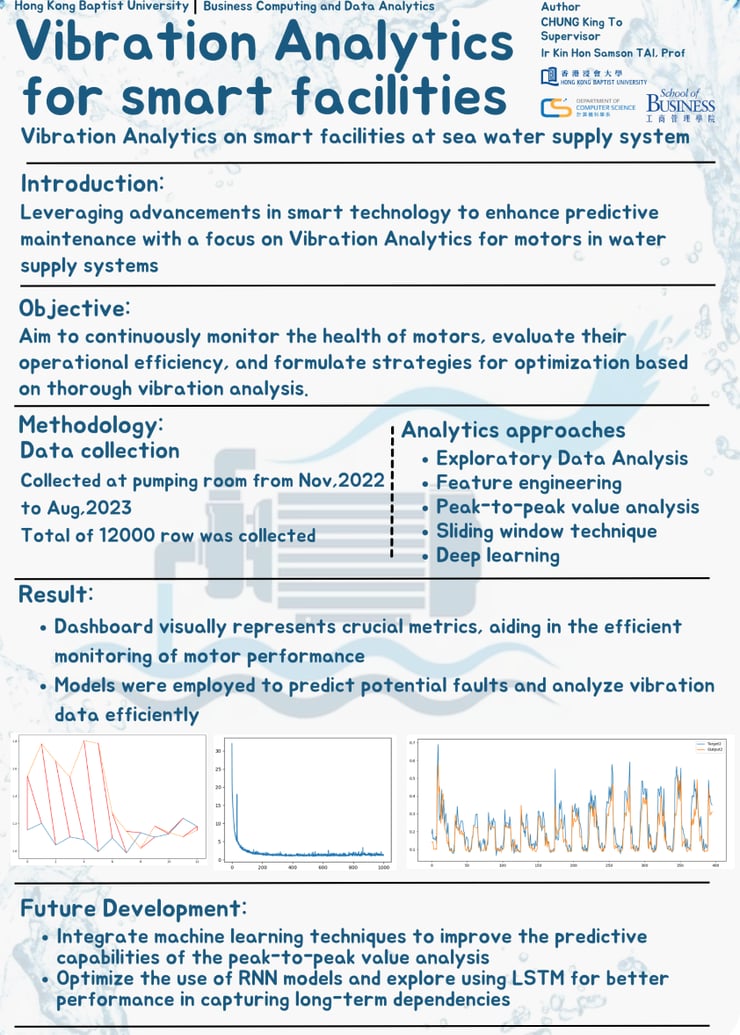
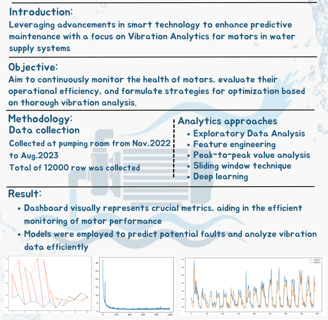